Back
Detection of thalassemia from peripheral blood smears using AI
การตรวจเม็ดเลือดที่ผิดปกติในผู้ป่วยธาลาซีเมียโดยการใช้ AI
@คณะแพทยศาสตร์
#KLLC 2024
#Healthcare and Wellness
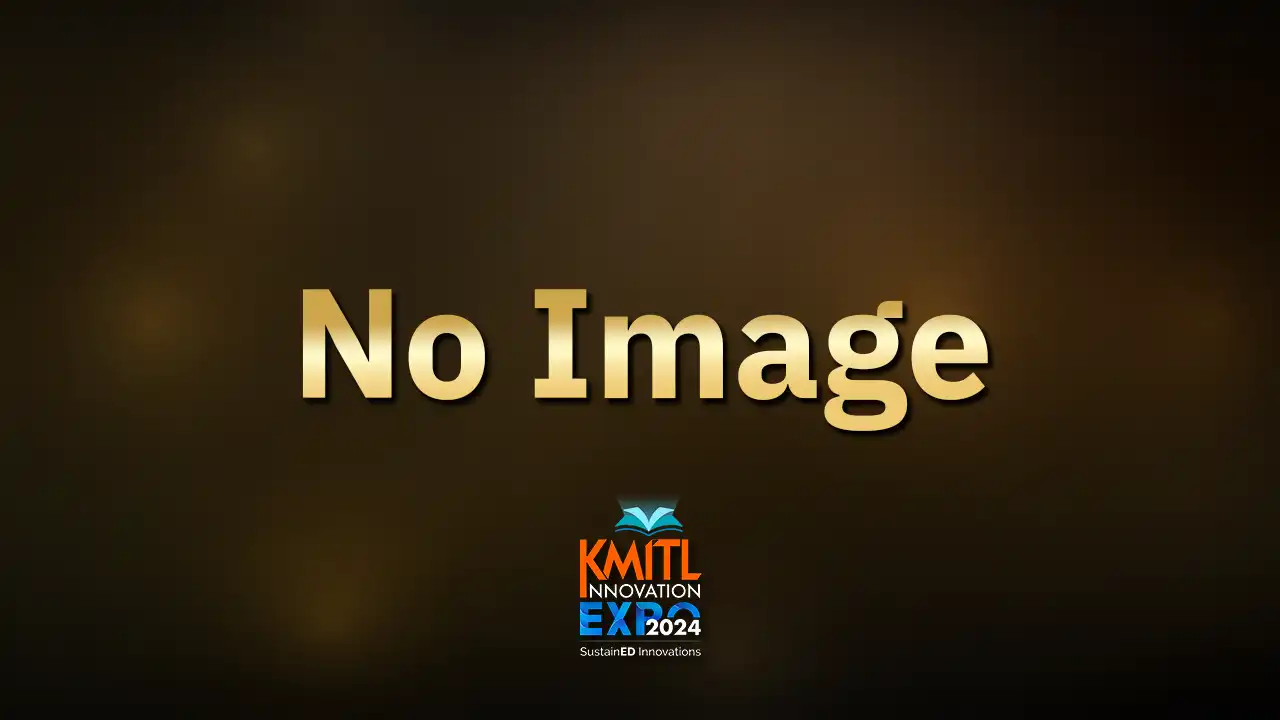
Details
This study aims to develop an AI-driven model capable of identifying abnormal red blood cells (RBCs) associated with thalassemia in a peripheral blood smear. The model's task involves distinguishing thalassemia from peripheral blood smears by categorizing white blood cells (WBCs) and six different types of RBCs including normal, abnormal, nucleated RBCs, spherocytes, cells with inclusion bodies, and target cells. The dataset, consisting of seven-categories with multiple-objects in each, was randomly split into two sets: a training- and a testing-set containing 100- and 20-images, respectively. The development process involved the creation of concatenated-models using You Only Look Once (YOLO) neural networks, including YOLOv4-128, YOLOv4-256, and YOLOv4-tiny.
Objective
Thalassemia, the most prevalent inherited-blood disorders, particularly in Southeast Asia, leads to anemia due to genetic defects affecting the production of globin proteins. To diagnose it accurately, conventional- and standard complete blood count (CBC) methods, which are time-consuming and dependent on hematological experts, are required. Moreover, they are susceptible to inter-examiner variability. To address these challenges, there is a growing need for innovative automatic screening tools. Recent developments in medical research, incorporating artificial intelligence (AI), have shown promise and indicate a future trend toward the integration of cutting-edge technology in thalassemia diagnosis.
Project Members
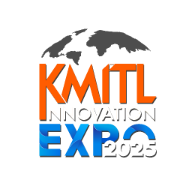
กมลชนก พนิชย์สกุล
KAMOLCHANOK PANICHSAKUL
#นักศึกษา
Member
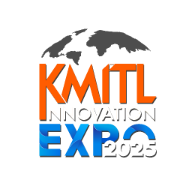
คณิศร ทองพิทักษ์ถาวร
KANISORN THONGPITUKTHAVORN
#นักศึกษา
Member
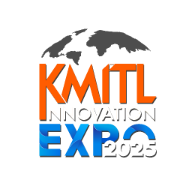
สิริน ริ้วเหลือง
SIRIN RIEWLUANG
#นักศึกษา
Member
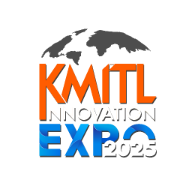
วีรยุทธ กิตติชัย
Veerayut Kittichai
#อาจารย์
Advisor
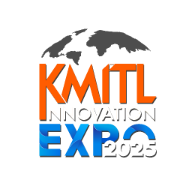
วีระฉัตร สมพงษ์
Weerachat Sompong
#อาจารย์
Co-advisor
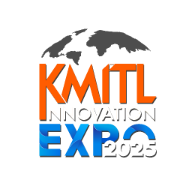
ศิริเดช บุญแสง
Siridech Boonsang
#อาจารย์
Co-advisor
Vote for this Innovation!
Loading...
Powered By KMITL Innovation Project