Back
SEMANTIC BIRD’S-EYE-VIEW MAP PREDICTION USING HORIZONTALLY-AWARE PYRAMID OCCUPANCY NETWORK
การทำนายภาพแผนที่มุมสูงโดยใช้แบบจำลอง HORIZONTALLY-AWARE PYRAMID OCCUPANCY NETWORK
@คณะเทคโนโลยีสารสนเทศ
#KLLC 2024
#Digital Technology
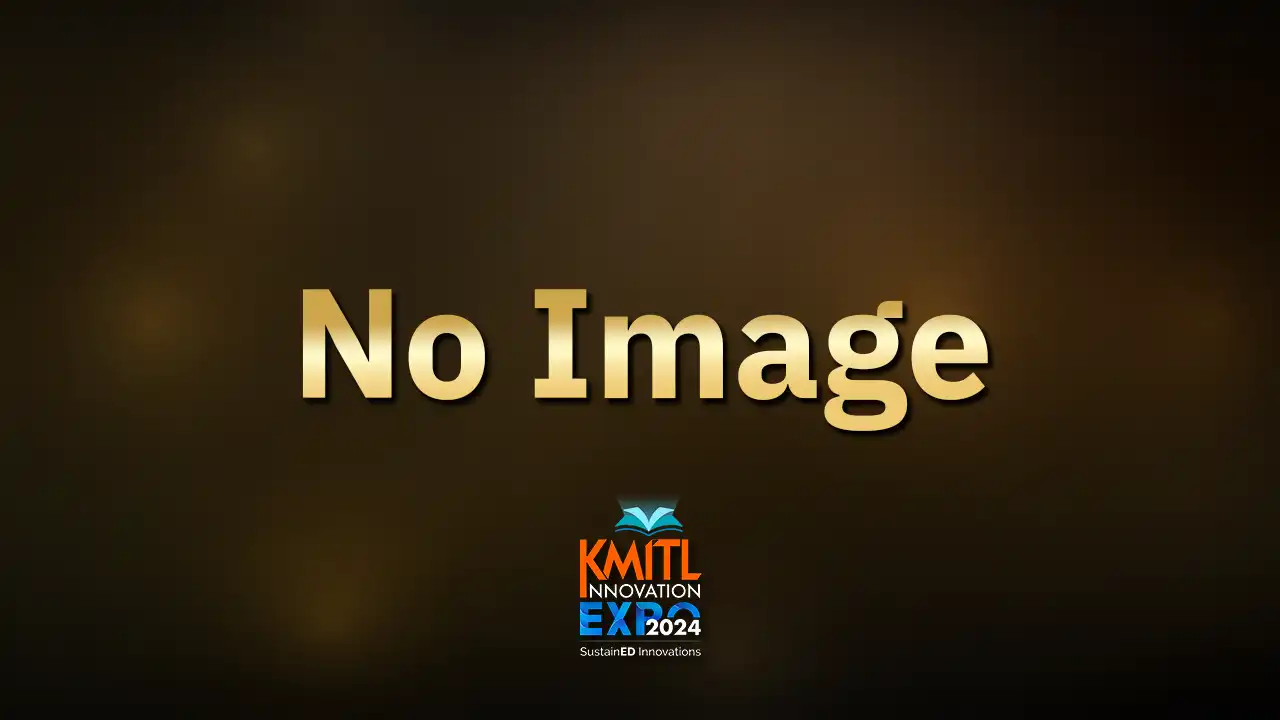
Details
Deep neural network has been used to predict the bird's-eye-view map from a frontal camera of an autonomous car. A state-of-the-art approach, namely pyramid occupancy network (PON), uses an encoder-decoder architecture to condense an image column into a context vector that describes the object occupancy along the radial direction. Our work, Horizontally-aware Pyramid Occupancy Network (H-PON), extends the PON model with a novel component that provides additional context information describing the relationships of the objects along the horizontal direction. This is done by also encoding the horizontal column of the image into an additional context vector using another encoder-decoder layer. This context vector is, then, expanded back providing improved features for semantic reasoning across the horizontal direction. We found that this simple extension significantly improves PON's semantic prediction performance in the nuScence dataset. Our experiment shows that the objects that are rarely seen and those that are further away from the center greatly benefit from this novel component.
Objective
With the advancement of technology, the automotive industry has shifted its focus from mere transportation to prioritizing convenience and safety. This has led to the emergence of autonomous driving, which brings benefits to both road users and passengers. Autonomous driving encompasses various components, ranging from understanding the surrounding environment to achieving self-driving capabilities. To sense and perceive the environment, autonomous driving heavily relies on object detection, distance estimation, and position estimation, which are achieved through a combination of lidar, radar, and cameras. However, lidar has drawbacks such as its weight and cost, while cameras offer a more affordable alternative that can perform these tasks without the need for lidar and radar. Top-down maps play a crucial role in autonomous driving as they provide a comprehensive bird's-eye view of the environment. These maps contain contextual information that aids autonomous driving systems in making well-informed decisions, especially in terms of efficient path planning by analyzing road layouts. The contextual information derived from top-down maps enables autonomous vehicles to identify and analyze potential obstacles in their surroundings. In summary, the objective of this work is to develop a deep learning model for autonomous driving that can predict semantic maps from images to reduce the cost associated with lidar and radar sensors.
Project Members
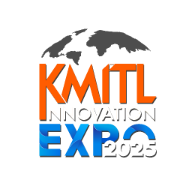
ตุลธร วงศ์ชัย
TUNLATON WONGCHAI
#นักศึกษา
Member
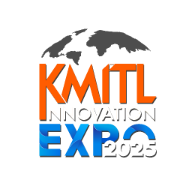
ธนภัทร ธีรรัตตัญญู
THANAPAT TEERARATTANYU
#นักศึกษา
Member
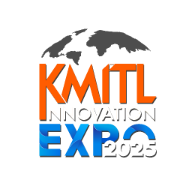
ณัฏฐ์ ดิลกธนากุล
Nat Dilokthanakul
#อาจารย์
Advisor
Vote for this Innovation!
Loading...
Powered By KMITL Innovation Project