Back
MOBILE APP FOR RETINAL DISEASE CLASSIFICATION USING DEEP LEARNING
แอปมือถือสำหรับการจำแนกโรคจอประสาทตาโดยใช้ Deep Learning
@คณะวิศวกรรมศาสตร์
#KLLC 2024
#Healthcare and Wellness
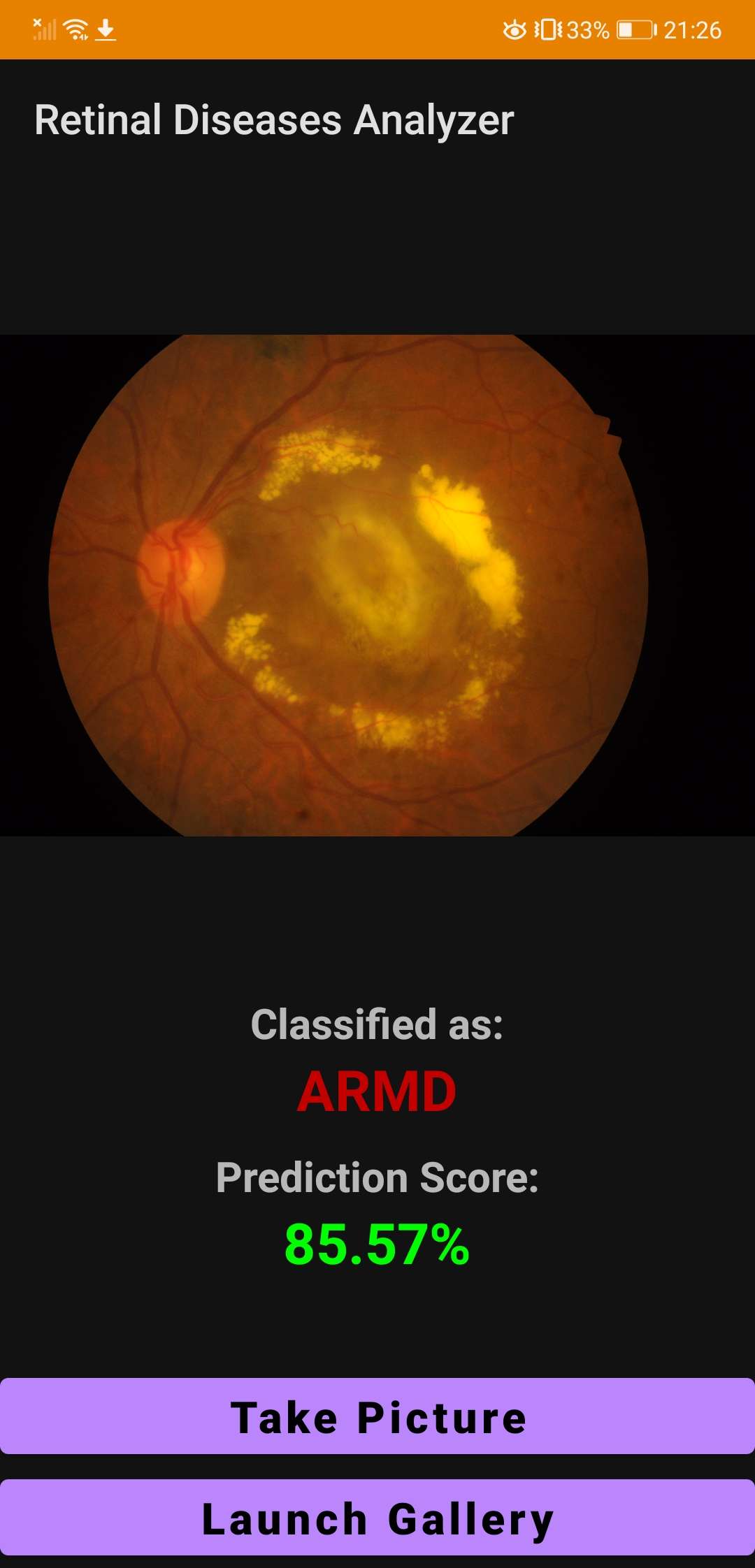
Details
A common retinal illness can have severe consequences for eyesight and eye health. Diabetic retinopathy is an example of a retinal illness that affects the blood vessels in the retina and can result in visual impairment or blindness. Early discovery, adequate treatment, and regular monitoring are critical in controlling these diseases and minimizing their effects on vision. An automatic classification system for detecting retinal illness using optical coherence tomography (OCT) and non-mydriatic camera pictures was developed using the MobileNetV2 deep learning network. With a sample size of 224 pixels, the scans are separated into eleven classes — Age-related macular degeneration (ARMD), branch retinal vein occlusion (BRVO), drusens (DN), diabetic retinopathy (DR), media haze (MH), myopia (MYA), optic disc cupping (ODC), optic disc edema (ODE), optic disc pallor (ODP), tessellation (TSLN), and normal retina (Normal). It is straightforward to convert models from MobileNetV2 into Android mobile apps for point-of-care diagnostics. The model was updated using validation samples, and its accuracy and sensitivity were evaluated. The model's performance was evaluated using measures like accuracy, precision, recall, and F1-score. According to the results of experiments on a large dataset, the suggested MobileNet V2 has an accuracy of 0.9575 for training, 0.9470 for validating, and 0.9630 for testing. Precision, recall, and F1-score values were also obtained, demonstrating high performance in retinal disease categorization.
Objective
การวิเคราะห์ภาพทางการแพทย์เป็นวิธีการวินิจฉัยโรคทางตาแบบดั้งเดิมที่ใช้เวลานาน ความก้าวหน้าล่าสุดในการเรียนรู้เชิงลึกและปัญญาประดิษฐ์ (AI) ช่วยให้เกิดการพัฒนาระบบการวินิจฉัยโดยใช้คอมพิวเตอร์ช่วย (CAD) ซึ่งสามารถปรับปรุงความแม่นยำและความเร็วในการตรวจหาโรคจอประสาทตาได้ ในการวิจัยนี้ จะมุ่งเน้นไปที่การพัฒนาวิธีการจำแนกประเภทอัตโนมัติสำหรับการระบุโรคจอประสาทตาจากภาพถ่ายจากกล้องที่ไม่ใช่ม่านตาและภาพเอกซเรย์เชื่อมโยงกันด้วยแสง (OCT) นอกจากนี้ จะมีการดำเนินการตรวจสอบเพื่อพิจารณาความเป็นไปได้ในการสร้างแอปมือถือ Android สำหรับการวินิจฉัย ณ จุดดูแลโดยใช้แนวทางที่แนะนำ โดยสรุป วัตถุประสงค์สูงสุดของการวิจัยนี้คือการฝังโมเดล deep learning ลงในแอปพลิเคชันสมาร์ทโฟน Android ที่สามารถตรวจจับความเจ็บป่วยของจอประสาทตาได้อย่างรวดเร็วและถูกต้องโดยใช้การสแกนจอประสาทตา โปรแกรมนี้ใช้เครือข่ายการเรียนรู้เชิงลึก MobileNetV2 เพื่อจัดหมวดหมู่ความผิดปกติของดวงตาจากการสแกนจอประสาทตา เพื่อปรับปรุงความเร็วและความแม่นยำของการวินิจฉัยปัญหาสายตา ซึ่งนำไปสู่ผลลัพธ์ของผู้ป่วยที่ดีขึ้นในท้ายที่สุด
Project Members
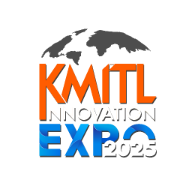
ภัทรกร อินทรประสิทธิ์
PATTARAKORN INTARAPRASIT
#นักศึกษา
Member
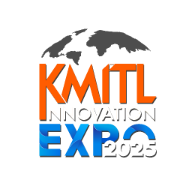
May Phu Paing
May Phu Paing
#อาจารย์
Advisor
Vote for this Innovation!
Loading...
Powered By KMITL Innovation Project